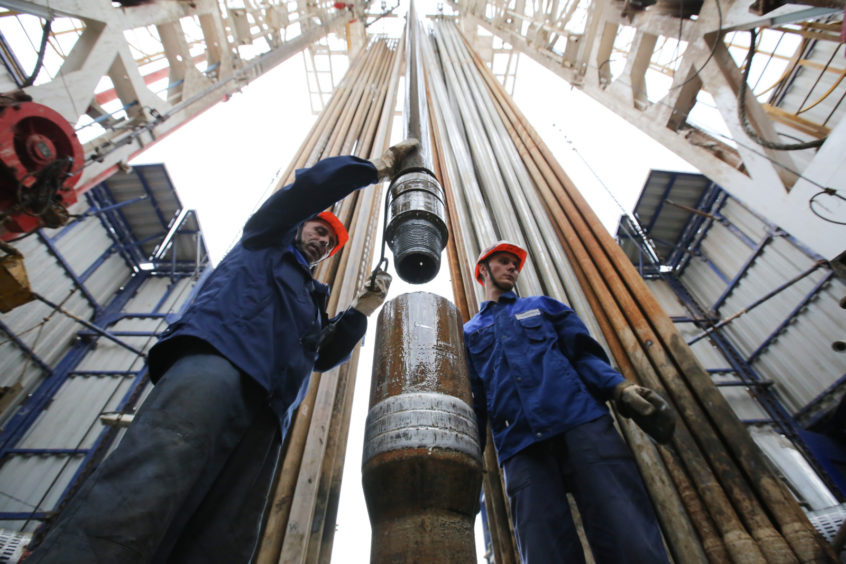
Meta: In the oil and gas industry, Industrial Internet of Things-driven solutions can increase the productivity of drilling and extraction operations by 30% by turning the data from multiple geographically distributed oil wells into informative insights about wells’ performance.
How Upstream Oil and Gas Benefits from IIoT
EY reports that the operational efficiency in the upstream segment has been steadily declining at the rate of 11% a year over the past four years. According to the same report, one of the reasons behind the reduction in the segment efficiency is poor well performance management, which can be traced back to the O&G personnel not having access to oil well data. In fact, it was estimated that only 1% of the information collected from the wells is made available to the O&G decision makers.
Today, IIoT comes to the upstream segment’s rescue and offers a more elaborate approach to managing and analyzing the data from wells. In this article, we’ll provide a closer look at how IIoT enhances the upstream segment’s performance with the focus on drilling and extraction operations.
Where IIoT fits in
Traditionally, SCADA has been an upstream segment’s backbone for managing well performance data. However, even though SCADA allows conducting basic visualization of well data and analyzing streaming data on the fly, it fails to provide enough storage capacity and advanced analytics capabilities for historical operational data from numerous geographically distributed sites, while the insights derived from this data are critical for increasing the segment’s performance.
IIoT fills in these gaps in SCADA’s functionality and provides opportunities for storing and performing advanced analytics on historical data from a number of geographically distributed wells, which promises to boost crude oil output by as much as 10% over a two-year period.
IIoT: What it is and how it works
Monitoring the effectiveness of drilling and extraction operations boils down to keeping an eye on the utilization of relevant equipment in the first place. To enable that, IIoT works in coordination with SCADA, the latter being a source of equipment operational data for the IIoT systems. In some cases, however, when SCADA cannot provide enough of the required data, the operational metrics can be fetched directly from sensors (e.g., proximity, vibration, torque) attached to equipment components. Once the data is collected, it is relayed over to the cloud platform – a core of an IIoT solution – for storing and analysis.
Along with sensor readings and the data obtained from SCADA, the cloud platform stores meta data (e.g., the information about the locations, calibrations of sensors), and the data fetched from other enterprise systems (e.g., equipment maintenance data, geophysical data). The advanced analytics component of IIoT analyzes this data for hidden patterns, which cannot be detected with traditional analytics methods. For that, the aggregated data set is run through machine learning (ML) algorithms. As a result, machine learning models are created. The models are applied to carry out a range of non-trivial tasks: predict the productivity of drilling and extraction operations depending on external conditions (e.g., rock permeability, injection rate and temperature), identify operational issues hindering equipment performance (e.g., kicks, washouts), and develop best practices for improving drilling and extraction processes productivity.
Drilling equipment monitoring
To provide drilling specialists with a view into onshore drilling rigs’ performance, the analytics component of an IIoT solution analyzes such drilling process parameters as draw works rotation frequency, a downhole fluid flow rate, standpipe and pump manifold pressure, top drive rotary torque and hook load. Once analyzed, these metrics provide insights into such performance indicators as the drilling depth, the rate of penetration (ROP), weight-to-weight connection time, surface and bottomhole pressure, torque on bit, and weight on bit. The analysis of the indicators’ values against contextual data (e.g., rock permeability) helps to prevent such issues as kicks, washouts, and loss of circulation, all directly affecting the productivity of the drilling operations.
An even larger value of applying IIoT for drilling optimization comes from its ability to carry out advanced analysis of the historical rig data. Combining the data about several downhole parameters (rate of penetration, torque on bit, mechanical specific energy) with the context (geophysical data) and running the combined data set through machine learning (ML) algorithms allows accurately representing downhole conditions and finding non-obvious correlations among the downhole parameters’ values. The uncovered correlations are reflected in ML models. Applied to the streaming drilling data, the models identify downhole parameter combinations that allow achieving highest ROP in given geophysical conditions, and recommend optimal equipment calibrations to achieve drilling targets. Moreover, the ML models may be used as real-time drilling advisory systems, recognizing drilling risks in real time and notifying drilling personnel via web or mobile applications.
Extraction equipment monitoring
In the extraction segment, IIoT can be applied to monitor the performance of artificial lift systems, including sucker rod pumps and electrical submersible pumps (ESPs).
Sucker rod pumps
The performance of sucker rod pumps can be monitored with the help of sensors that collect data about downhole pressure and speed of a motor. Once analyzed, these parameters provide a look into the fluid level in the pump chamber and the rate at which the pump produces. At the same time, as the entry of gas into a pump can significantly reduce the rate at which the pump produces, gas detectors are additionally deployed to monitor the contents of gas in the pump chamber.
One of the areas where advanced analytics is applied is the analysis of pumps’ dynamometer cards, which provides insights into pumps’ operating parameters (e.g., pumping unit speed, rod position) and performance. To provide these insights, the cards have to be interpreted. Manual interpretation of a pump card is a labor-intensive process that requires a lot of time and deep domain expertise. As oil fields generate more and more data, it becomes harder for humans to interpret it. Machine learning-based pattern recognition automates card interpretation process and allows proactively monitoring rod pumps’ performance.
Electrical submersible pumps (ESPs)
For monitoring the performance of ESPs, surface and downhole parameters are monitored. The key surface parameter to monitor is a total flow rate. Crucial downhole parameters include pump discharge and intake pressure, pump intake temperature, and motor oil temperature. Once analyzed, these parameters provide a view into ESP’s pull rates, surface and subsurface activity.
The advanced analytics component of IIoT allows analyzing the combined data set comprised of pumps’ historical operational parameters (gauged oil flow rate, periods of intermittent pump operations, pump vibration frequency) and contextual information to create ML models, which recommend optimal pump calibrations to provide for its stable operation and ensure maximum oil flow rate.
IIoT: to try or not to try
IIoT has some unique benefits that make it a good fit for the upstream industry, including:
• The possibility to analyze the data from multiple wells and rigs. Ingesting data from multiple geographically distributed reservoirs and extracting insights from the aggregated data helps to compare performance across the wells, develop best practices for reservoir stimulation and recovery optimization.
• The possibility to identify the root causes of equipment performance issues. Analyzing historical operational data against contextual information allows detecting factors catalyzing performance losses. Early identification of these factors helps upstream companies make data-driven decisions regarding the choice of the relevant drilling and extraction strategies.
However, to reap the promised benefits, before rolling out IIoT-driven solutions, upstream companies have to consider the following limitations:
• Legacy equipment should be connected to an IIoT solution in a way that ensures fast and reliable data transmission across TCP/IP network. Many legacy machines, however, were designed to communicate locally with SCADA and cannot be easily connected to the Internet via TCP/IP. Although there are physical gateways that can translate between legacy systems and newer protocols, the integration challenge is still to be resolved.
• Drilling rigs and extraction pumps often operate in hard-to-access locations with poor communication coverage. Possible disruptions in the communication can lead to some data entries not being available or available with delays, which can influence the analysis accuracy.
On a final note
The upstream oil and gas sector loses billions of dollars annually due to non-productive time. IIoT will be able to increase the segment’s efficiency by uncovering downtime root causes and enabling oil and gas professionals to proactively mitigate them. According to CERA, the adoption of IIoT-driven technologies in the upstream oil and gas is likely to cut production costs by up to 6%, reduce well downtime by up to 4%, and decrease the segment’s labor intensity by up to 25%.
Boris Shiklo, CTO at ScienceSoft, is responsible for the company’s long-term technological vision and innovation strategies. Under his supervision, the company’s development team has successfully fulfilled complex projects of over 80,000 man-hours in Healthcare, Banking & Finance, Retail, Telecommunications, Public Sector and other domains. Boris Shiklo has a solid background in IT consulting, software development, project management and strategic planning.