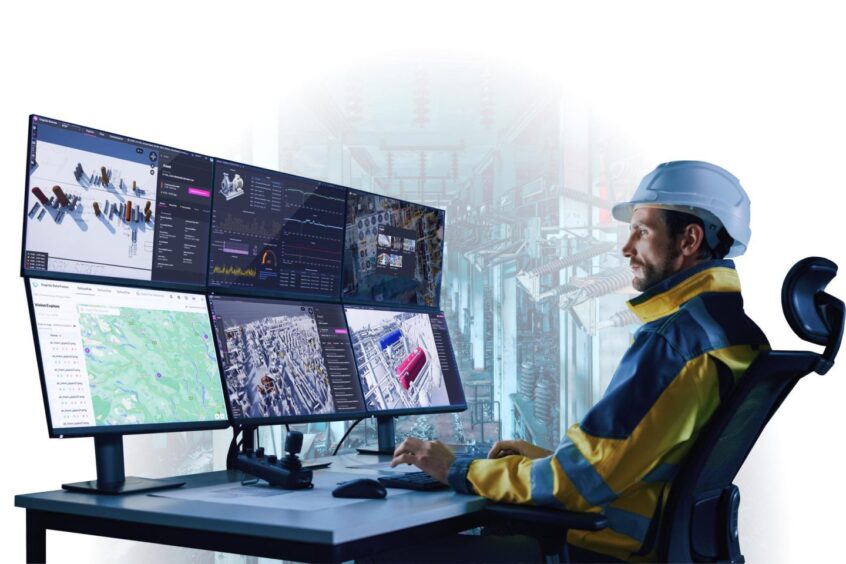
AI for the energy industry has arrived with a hefty promise to dramatically increase operational excellence and performance across the value chain. From doubling the efficiency of field inspections on FPSOs to helping address the risks of SME brain drain, industrial data and AI is already driving meaningful change.
But many operators are finding that there are two sides of this coin. While AI is accelerating digital transformation and delivering value faster than ever before, it is also creating more short-term complexity for industrial leaders that can be difficult to navigate and stall progress.
Some questions are getting harder
What is our overarching AI strategy? What operational use cases should be prioritised? What AI approach should we use for each use case? Is our data infrastructure even ready for an AI future? How should we organize to address the opportunity and maintain our competitive edge?
With varying degrees of AI-based autonomous operations now being the clear end goal, complete with millions of dollars of expected operational value and impact to EBIT, it’s easy to get lost in the nuance and complexity. Without clear data and AI-centric milestones, it’s actually very difficult to make sustained progress towards that end goal without setbacks, regrettable vendor choices, or promising, but unscalable proofs of concept.
The good news? There’s a playbook that addresses these questions:
1) Repeatable data and AI success can be scaled down to concrete steps and milestones
2) The technological backbone for scaled use cases has never been more effective.
Get the modern AI playbook for industrial leaders
Pillar 1: Simple Access to Complex Industrial Data
Whether your digital team will admit it or not, most still have not conquered the industrial data problem due to the inherent complexity and messiness of industrial data. Historian, ERP, Work Order, EPC data and more should be accessible to everyone who has the right permissions without hours of data prep legwork or quality concerns. Solving this problem first with modern industrial data and AI tools unlocks immediate value in terms of smarter day-to-day operations and more effective RCA, troubleshooting, and more.
Pillar 2: Operational Use Cases at Scale
There are many more examples of individual proof-of-concept success across the energy industry than examples of scaled deployments across multiple assets or sites. Many operators presume adjusted per-unit economics when considering data onboarding, maintenance, and analytics that hurt the overall ROI of their project, even if the initial phase was considered a success. Modern data infrastructure uses AI and other automation to bring the man-hours required for scaling down by ~80%.
Pillar 3: Autonomous AI Agents
The industrial AI future doesn’t belong to a single AI co-pilot, rather multiple AI agents that work together to enable your experts and workflows with data and automation. From summarizing key information across multiple documents, to plotting asset health trends, to populating and maintaining data models, many AI agents will be developed by vendors and operators to improve daily workflows across the energy value chain. Are you equipped to deploy, support, and manage industrial AI agents with a modern workbench and approach?
Don’t miss the modern AI playbook for industrial leaders
What’s keeping you from making progress?
There’s still time this year to solve for one or two of the pillars in this playbook and minimize your risk of falling behind. With most of our projects delivering value in 6-8 weeks, Cognite is uniquely trusted by industry and positioned to help you succeed with data and AI capabilities built from industry, for industry.
What your peers are paying attention to: